How to use Al for data quality? : A Complete Guide
- Gunashree RS
- Apr 8
- 5 min read
🔍 Introduction: Why AI and Data Quality Are a Perfect Match
In today’s digital-first landscape, data is the backbone of every business decision. But the reality is harsh—most organizations suffer from poor data quality. Inaccuracies, duplicates, and missing values disrupt operations, analytics, and decision-making.
That’s where Artificial Intelligence (AI) enters the scene—not just as a buzzword, but a true game-changer. From automating data cleaning to predicting anomalies and enhancing consistency, AI empowers companies to improve data quality efficiently.
This guide will walk you through how to use AI for data quality—tools, techniques, benefits, challenges, and real-life use cases included.
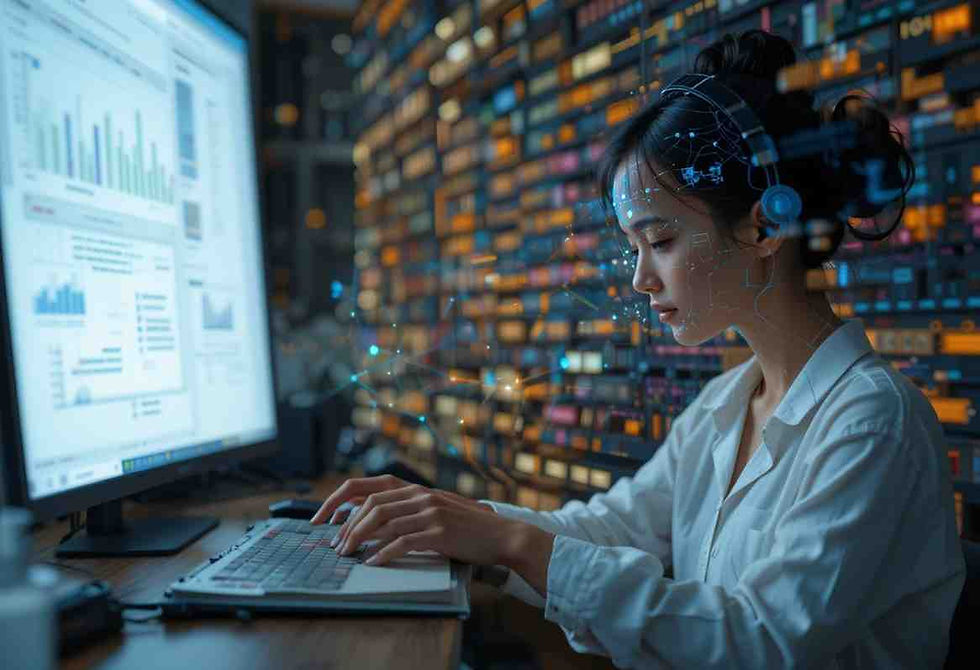
📊 Understanding Data Quality: Foundation First
Before diving into AI, it’s essential to understand what data quality means.
🔹 What is Data Quality?
Data quality refers to the accuracy, completeness, consistency, timeliness, and reliability of data used for decision-making.
🔹 Key Dimensions of Data Quality
Accuracy – Data reflects the real-world scenario.
Completeness – No critical data is missing.
Consistency – Uniform formatting across systems.
Timeliness – Data is up to date.
Validity – Conforms to rules and constraints.
🔹 Challenges Without AI
Manual data entry errors
Siloed systems and redundant data
Slow cleansing and validation processes
Lack of real-time insights
🤖 The Rise of AI in Data Management
AI’s journey in data management started with basic automation but has evolved into sophisticated algorithms capable of understanding, interpreting, and enhancing data in real time.
Major Catalysts of AI Adoption in Data Quality:
Growth of big data
Need for real-time decision-making.
Regulatory compliance pressure
Demand for clean, actionable insights
💡 Benefits of Using AI for Data Quality
AI dramatically transforms data quality efforts. Here's how:
Speed: Real-time cleansing and validation
Accuracy: Reduces human errors
Consistency: Applies rules uniformly across datasets
Scalability: Handles large datasets effortlessly
Predictive: Detects potential data issues before they occur
🛠️ AI Techniques for Data Quality Management
Machine Learning (ML)
Identifies data patterns
Learns from past data corrections
Flags anomalies intelligently
Natural Language Processing (NLP)
Cleanses textual data
Extracts meaning from unstructured data
Anomaly Detection Algorithms
Spots outliers in numeric and text data
Useful in fraud detection, pricing errors
📁 Data Profiling with AI
AI can automatically scan datasets to generate insights like:
Data types and formats
Missing values
Frequency distributions
Pattern identification
This helps build robust data quality rules without human intervention.
🧹 Data Cleansing and Enrichment with AI
AI can:
Detect and remove duplicate records
Correct typos using ML suggestions
Enrich data by filling in missing attributes (like ZIP codes or company names)
Standardize inconsistent entries (e.g., converting all dates to MM/DD/YYYY format)
🔗 AI for Data Matching and Integration
AI enables:
Entity Resolution: Identifying if "John A. Smith" and "J. Smith" are the same person
Record Linkage: Merging data from different sources into a single view
It uses ML models trained on previous matches to improve over time.
📡 Real-Time Data Monitoring Using AI
With AI:
Set up real-time dashboards
Receive alerts for invalid data entries.
Automatically trigger workflows for correction.
This creates a self-healing data pipeline.
🧠 Using NLP to Improve Textual Data Quality
AI-powered NLP can:
Analyze customer reviews for sentiment
Normalize language (e.g., changing "u" to "you")
Detect inappropriate or irrelevant content.
📂 AI for Metadata Management
AI can help auto-generate metadata such as:
Column definitions
Source lineage
Usage statistics
This boosts data discoverability and cataloging efforts.
📜 Governance and Compliance with AI
AI supports:
Automated audits
Regulation mapping (GDPR, HIPAA)
Privacy risk detection in datasets
⚠️ Challenges in Using AI for Data Quality
Even with its potential, AI isn’t without obstacles:
Data Bias: Models learn from historical bias
Transparency: It is hard to explain ML decisions
Implementation Costs: Requires skilled talent and infrastructure
📌 Best Practices to Implement AI for Data Quality
Define Clear Objectives – Know what you want AI to fix
Start Small – Use a pilot project.
Use Quality Datasets – Train AI models on trusted data.
Monitor Continuously – Set up alerts and metrics.
Combine Human and AI Efforts – Use AI as an assistive tool.
🧰 Top Tools and Platforms for AI-Driven Data Quality
Tool | Use Case |
IBM InfoSphere | Data cleansing & profiling |
Talend Data Fabric | End-to-end data quality |
Informatica | AI-powered metadata management |
Trifacta (Google Cloud) | Data wrangling |
Ataccama ONE | Centralized data quality with AI |
🏥 Industry Applications of AI for Data Quality
Healthcare
Detect duplicate patient records
Normalize medical terminologies
Finance
Validate KYC information
Spot fraudulent transaction patterns
Retail
Clean customer segmentation data
Enrich product catalogs
Logistics
Match vendor details
Optimize delivery data with clean geocodes
📈 Measuring ROI from AI in Data Quality Projects
Key Metrics:
% reduction in errors
% increase in automated corrections
Time saved in manual processes
Cost savings in operations
Business impact (faster decisions, more sales)
🔮 Future of AI in Data Quality
Self-healing datasets
Zero-touch data pipelines
Federated AI for privacy-respecting learning
AI copilots for data teams.
The future is bright—and it’s automated.
🙋♂️ Frequently Asked Questions (FAQs)
Q1: How does AI detect bad data?
AI uses machine learning algorithms to identify patterns, detect anomalies, and compare with known valid values.
Q2: Can AI automatically fix data errors?
Yes, many tools allow AI to auto-correct typos, duplicates, and formatting issues based on training data and rules.
Q3: Is AI better than manual data cleansing?
AI is faster, scalable, and more consistent. However, a human-in-the-loop approach is still valuable for complex decisions.
Q4: What are some real-life AI data quality examples?
Healthcare systems use AI to clean patient records, while banks use it to validate customer data during onboarding.
Q5: What are the best AI tools for data quality?
IBM InfoSphere, Talend, Informatica, and Trifacta are among the top tools for AI-driven data quality management.
Q6: Is using AI for data quality expensive?
While initial setup can be costly, it saves long-term expenses by automating data tasks and reducing error-related losses.
Q7: Can AI help with regulatory compliance?
Yes, AI tracks data usage, detects PII, and ensures rules like GDPR or HIPAA are followed automatically.
Q8: What industries benefit most from AI in data quality?
Healthcare, finance, retail, and logistics see the biggest benefits due to large volumes of sensitive, structured, and unstructured data.
🧾 Conclusion: How to use Al for data quality?
Clean data is the fuel for smart decisions, and AI is the engine that makes it happen. With the right approach, tools, and governance, AI transforms your messy datasets into accurate, trusted assets that drive growth, efficiency, and innovation.
Don't just collect data—empower it with AI.
✅ Key Takeaways
AI enhances accuracy, consistency, and speed in data quality tasks
Machine learning and NLP are key techniques in cleansing and profiling.
Real-time monitoring and data matching are simplified with AI tools.
Compliance, governance, and auditing benefit from AI automation.
Top tools include Talend, Informatica, and IBM InfoSphere.
Industries like healthcare and finance gain major advantages.
Start small, monitor continuously, and combine AI with human oversight.
Comentarios