What is Quality AI? Powerful Guide + Key Insights
- Gunashree RS
- 2 days ago
- 4 min read
Introduction to Quality AI
Artificial Intelligence (AI) is revolutionizing every corner of modern life—from self-driving cars to virtual assistants. But amid this rapid growth lies a crucial question: What is quality AI? In simple terms, it’s not enough for AI to be powerful—it must also be accurate, ethical, transparent, and beneficial for humanity.
As organizations race to integrate AI into operations, the term “quality AI” emerges as a benchmark for safe, reliable, and responsible AI systems. It’s about creating models that not only work but work well—with minimal bias and maximum fairness. This guide offers a deep dive into what quality AI entails, its significance, how to measure it, and the road ahead.
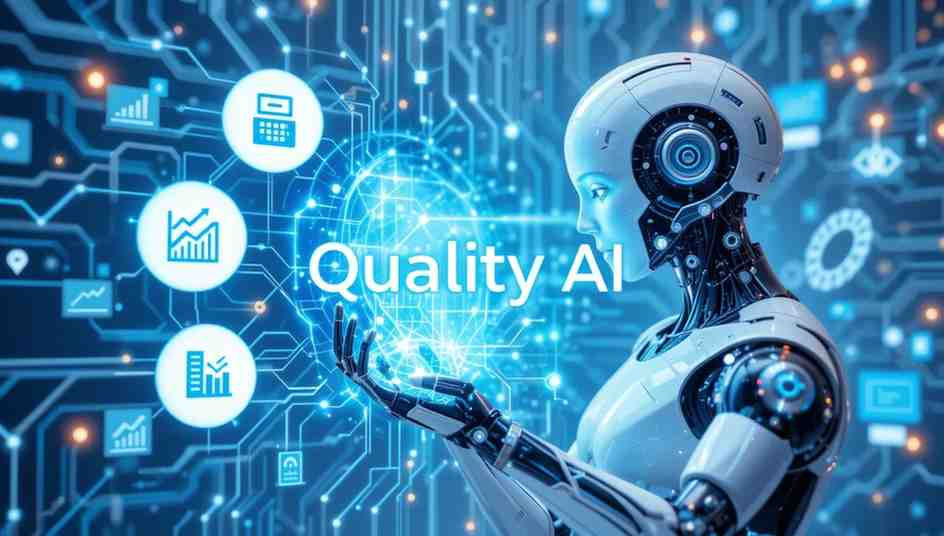
Defining “Quality AI”
What Sets Quality AI Apart?
Quality AI refers to systems designed and deployed with a deliberate focus on trust, fairness, transparency, and usability. While many AI systems aim for efficiency or automation, quality AI centers on human impact, ethical integrity, and sustainable outcomes.
Dimensions of AI Quality
Technical Soundness – Proper algorithm design, testing, and optimization.
Data Integrity – High-quality, unbiased, and diverse data sets.
User Trust – Confidence in AI's predictions and decisions.
Regulatory Compliance – Alignment with ethical and legal standards.
Core Characteristics of Quality AI
Accuracy
The foundation of AI quality lies in its ability to make correct predictions. Accuracy determines how close the model's outputs are to real-world values.
Reliability
AI systems must be consistent. A model that performs well only in test environments but fails in real-world scenarios lacks reliability.
Fairness and Ethics
AI must not favor one group over another. Mitigating algorithmic bias is a key marker of quality.
Interpretability
The AI’s decision-making process should be understandable. Black-box AI systems often fall short on this front.
Key Technologies Behind Quality AI
Machine Learning & Deep Learning
These drive the intelligence of AI. Quality depends on:
Model architecture
Training data volume
Hyperparameter tuning
NLP & Computer Vision
Used in applications like chatbots or facial recognition, NLP and CV must be tested for edge cases to ensure real-world applicability.
Quality AI vs Traditional AI
Comparative Overview
Aspect | Traditional AI | Quality AI |
Focus | Functionality | Functionality + Integrity |
Transparency | Often opaque | Explainable |
Fairness | May include biases | Actively mitigates bias |
Ethics | Rarely considered | Central to design |
Benefits of Prioritizing Quality
Higher user trust
Better ROI
Reduced legal/regulatory risks
Metrics for Evaluating Quality AI
Performance Metrics
Precision – How many of the predicted positives were truly positive?
Recall – How many of the actual positives were caught?
Human-Centered Metrics
User satisfaction
Understandability
Accessibility
Why Quality AI is Important Today
AI is everywhere – From hospitals to hiring systems.
Consequences are real – Misdiagnoses or wrongful denials can have life-altering effects.
Consumer awareness is rising – People demand transparency and fairness from tech.
Common Challenges in Achieving Quality AI
Data Bias: Poor or skewed data can lead to discriminatory models.
Explainability: Complex models like deep neural networks are hard to interpret.
Model Drift: Over time, models can lose accuracy as data patterns change.
The Role of Data in Quality AI
Garbage in, garbage out: The quality of AI is directly proportional to the quality of data.
Labeling accuracy: Human errors during annotation can derail outcomes.
Data diversity: To generalize well, models need training data from various groups.
Human-Centered Design in Quality AI
Humans-in-the-loop (HITL) can correct AI errors in real-time.
Usability testing ensures AI systems are user-friendly, especially for non-technical users.
Ethics and Regulation in Quality AI
Governments worldwide are proposing AI bills of rights, including transparency and accountability mandates.
Companies must integrate ethical audits to ensure compliance with GDPR, HIPAA, or other frameworks.
Industry Use Cases of Quality AI
Healthcare
Diagnosing diseases with transparent ML models
Risk predictions with explainable AI
Finance
Fair loan approvals
Transparent credit scoring
E-Commerce
Ethical recommendation engines
Chatbots with emotional intelligence
Education
Personalized learning without bias
AI tutors that adapt fairly to all students
Tools and Frameworks for Building Quality AI
TensorFlow and PyTorch for development
MLflow and Weights & Biases for tracking
Fairlearn, SHAP, and LIME for fairness and interpretability
How to Implement Quality AI in Projects
Define ethical goals.
Gather clean, diverse data.
Choose interpretable models when possible.
Test for fairness and drift regularly.
Keep humans in the feedback loop.
The Future of Quality AI
Explainable AI (XAI) will become the norm.
Regulations will shape product design.
AI will increasingly partner with human cognition rather than replace it.
Frequently Asked Questions
1. What is quality AI?
Quality AI is artificial intelligence that meets technical performance standards while being ethical, fair, transparent, and trustworthy.
2. How is quality AI measured?
By evaluating performance metrics (e.g., accuracy, recall) and ethical criteria like bias, fairness, and interpretability.
3. Why is quality important in AI?
Because poor AI can cause real harm—financial, reputational, or even physical.
4. What tools help build quality AI?
TensorFlow, PyTorch, Fairlearn, LIME, and data monitoring platforms.
5. What role does data play in quality AI?
Data quality and diversity are foundational to model performance and fairness.
6. Can AI be both powerful and ethical?
Yes, with deliberate design and continuous evaluation, AI can be both powerful and ethically sound.
Conclusion
The conversation around “What is quality AI?” is more than just academic—it’s foundational for the future of technology and society. As AI continues to integrate into daily life, ensuring that it is reliable, fair, and interpretable becomes non-negotiable.
Building quality AI is not just about better tech—it's about building a better world.
Key Takeaways
Quality AI is defined by accuracy, ethics, and user trust.
Transparent and fair AI systems build stronger public confidence.
Tools like SHAP and Fairlearn help developers build responsibly.
Bias in data and design can derail even the smartest AI models.
Human-centered design is crucial for long-term success.
Regulation and ethical frameworks are shaping AI’s future.
Lapak7d
Daftar Lapak7d
Login Lapak7d
Slot Gacor Terpercaya
Slot Toto 4D
Situs Slot Online
Slot Toto
Situs Resmi
Lapak7d
Situs Slot Resmi
Situs Slot Terpercaya
Slot Gacor
Situs Slot Online
Lapak7d
Situs Slot Terpercaya
Slot Qris
Situs Slot Online
Slot Gacor
Slot Pulsa
Situs Slot Indonesia
Link Slot Gacor
Lapak7d
Login Lapak7d
Daftar Lapak7d
Situs Slot Online
Situs Gacor
Slot Gacor
Situs Terpercaya
Slot Toto
Situs Slot Indonesia